Machine Learning in action: Real-world use cases
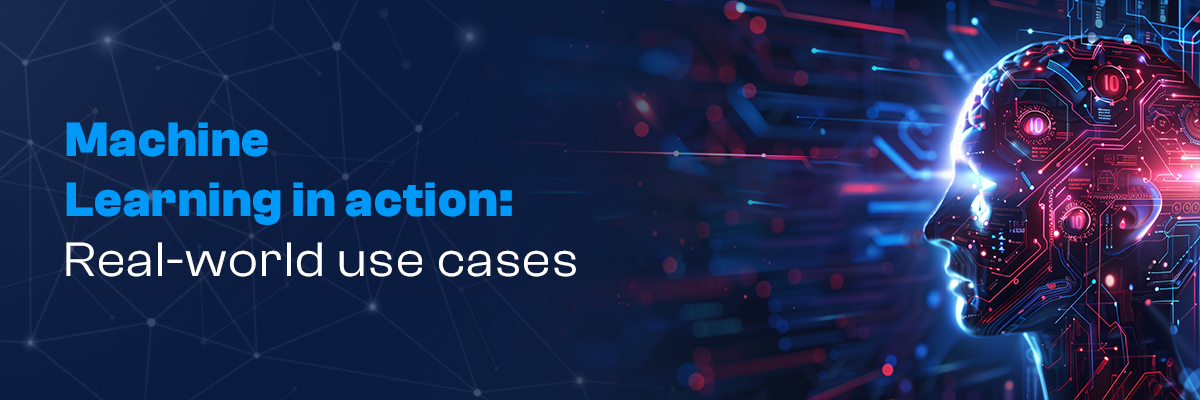
In recent years, machine learning (ML) has transformed from a theoretical concept into a core part of everyday business operations across various industries. With an impressive compound annual growth rate (CAGR) of 37.47%, the global ML market is set to skyrocket from USD 26.06 billion today to an astonishing USD 328.89 billion by 2031. This rapid expansion is fueled by breakthroughs in cloud computing, big data, and AI, pushing the boundaries of what machine learning can achieve.
The adaptability of machine learning enables it to drive an incredible spectrum of uses in sectors including marketing, finance, cybersecurity, and industry. Using ML, firms, including Amazon Web Services (AWS), Google, Microsoft, IBM, and others, are creating more intelligent systems, streamlining procedures, and offering individualised consumer experiences. This blog shows some of the most fascinating practical uses of machine learning, highlighting how this technology is transforming sectors.
Healthcare
Healthcare is undergoing a profound transformation with the help of machine learning (ML). Big data—including patient records, clinical trial data, and medical images—is becoming increasingly available. Therefore, ML systems can help with early disease identification, diagnosis, and tailored treatment strategies.
Predictive analytics is among the most prominent uses of machine learning in the medical field. Massive data analysis using ML models can find risk variables for disorders such as diabetes, heart disease, or cancer before they show clinically. This helps doctors create treatment strategies, therefore enhancing patient results.
Using ML-based prediction tools, for instance, a hospital can project patient readmissions and modify treatment regimens to reduce the likelihood of additional hospitalisation. Apart from improving patient care, this helps healthcare providers cut expenses.
Financial Risk Management and Fraud Detection
Banking has embraced machine learning, particularly in fraud detection and risk management. Traditionally, spotting fraudulent behaviour needed basic rule-based systems and hand reviews. However, as cyberattacks get more complicated, machine learning has become a necessary weapon against financial fraud.
Real-time transaction data analysis using machine learning systems can spot trends and anomalies suggesting possible fraud. This implies that banks and other financial institutions can flag dubious behaviour more precisely and far faster than in past times. Artificial intelligence-powered fraud detection systems have drastically cut fraudulent transactions, providing a safe space for customers and companies.
Another vital finance ability is risk management, which has also gained much from machine learning. Using analysis of a broad spectrum of elements, including credit history, market trends, and consumer behaviour, ML algorithms assist banks and financial institutions in assessing credit risk. This reduces losses and enhances financial planning by letting one make better-informed decisions on investments and loans.
Cybersecurity
One area where machine learning is causing waves is cybersecurity. Standard security measures are insufficient as cyber threats get increasingly complex. Improved by machine learning, AI-powered cybersecurity solutions are vital in preventing assaults using weak points in a company’s digital infrastructure.
The third-largest economy behind the United States and China, cybercrime is a primary concern worldwide, with losses predicted to surpass USD 6 trillion globally by 2021. By 2025, these numbers are expected to reach an astonishing USD 10.5 trillion. This urgent issue motivates the creation of sophisticated ML-based systems that can identify phishing attempts, ransomware, and real-time threat detectors.
Because machine learning models can always learn from fresh data, they can more successfully find and fix zero-day vulnerabilities—unknown software flaws—than conventional techniques. Two new artificial intelligence-driven cybersecurity solutions meant to improve corporate cyber resilience came from a recent partnership between Tata Consultancy Services and Google Cloud. These developments monitor dangers, spot deviations, and suggest fixes so companies can maintain a solid security posture before changing cyber threats.
Manufacturing
Machine learning finds application in manufacturing to maximise predictive maintenance and operations. Using previous data on equipment performance, ML techniques may forecast when a machine will likely fail, enabling manufacturers to perform maintenance before a breakdown. This raises general productivity and reduces expensive downtime.
Supply chains are being optimised using machine learning as well. ML models can assist companies in better resource allocation and operational streamlining decisions by analysing demand projections, inventory levels, and production schedules.
Retail
Understanding consumer behaviour has long piqued retailers’ curiosity, and machine learning provides robust instruments to help. Machine learning techniques can forecast what consumers will likely buy next, allowing stores to modify their marketing plans through data from prior purchases, web activity, and customer profiles.
Recommendation systems are one well-known instance of machine learning in retail. Using machine learning, companies like Amazon and Netflix propose goods or films depending on past behaviour. From fresh client interactions, these systems constantly learn and improve their recommendations to get more accurate.
Moreover, machine learning techniques can assist stores to maximise price policies. Analysing consumer demand, rival pricing, and market developments helps ML suggest ideal prices for goods, guaranteeing a balance between profitability and customer happiness.
Marketing
Machine learning is becoming indispensable in marketing for campaign optimization and client involvement enhancement. Analysing enormous volumes of data allows machine learning systems to spot consumer behaviour trends and suggest more likely successful marketing plans.
Machine learning, for example, can forecast which consumers are most likely to respond to a campaign or make a purchase, enabling companies to target these people with customised advertising. Cutting ineffective expenditures on non targeted efforts raises conversion rates and lowers marketing expenditures.
Machine learning also helps businesses do sentiment analysis—that is, to evaluate public opinion about a good or brand through customer comments, reviews, and social media postings. This enables companies to decide how to enhance their products and change their marketing plans.
Wrapping Up
As machine learning develops, its use in many sectors is growing more common and complex. From manufacturing and retail to healthcare and finance, machine learning can transform operations, improve decision-making, and enhance customer experiences. Forepected to reach USD 328.89 billion by 2031, the market provides evidence of its transforming ability.
Companies that use machine learning well in this digital era keep ahead of the curve and set the benchmark for creativity and efficiency. Machine learning is really in action—driving sectors forward and transforming the future of business—whether improving hospital care, safeguarding financial transactions, or optimising operations.